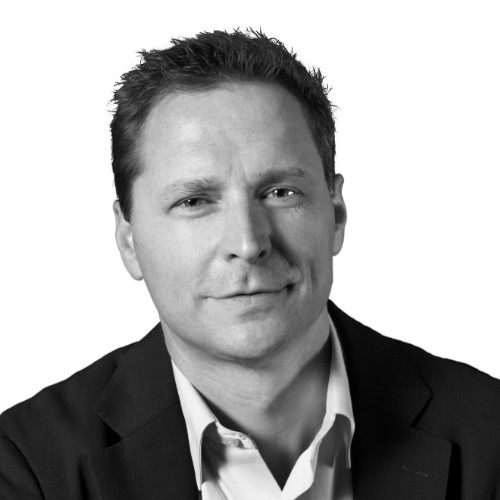
The digital economy, powered by sophisticated technologies like the Internet, mobile devices, cloud computing, and more, is experiencing a substantial shift due to the emergence of Artificial Intelligence (AI). These digital technologies have fueled the inception and growth of numerous economic activities. AI, with its capacity to analyze vast data sets, automate complex tasks, and drive innovation, is revolutionizing various aspects of the digital economy. Among AI’s multifaceted applications, recommender systems have emerged as a crucial tool that significantly impacts multiple industries, fostering user engagement, streamlining decision-making processes, and improving the efficiency of services.
Before diving into AI recommender systems’ intricacies, let’s ground our understanding with a real-world success story. NVIDIA’s triumph at the KDD Cup exemplifies AI’s prowess. Through the insights of NVIDIA partner Ronald van Loon, we’ll explore the essentials and challenges of AI recommender systems.
Case Study: NVIDIA’s Triumph at the KDD Cup
The KDD Cup, a prestigious competition organized by the Association for Computing Machinery’s Special Interest Group, provides a platform for contestants to solve real-world data science problems, including challenges related to recommender systems.
NVIDIA’s team emerged victorious in this prestigious competition by winning all three tasks for building state-of-the-art recommendation systems. This remarkable achievement by NVIDIA’s team highlights the potential and prowess of GPU-accelerated recommender systems in transforming the digital landscape. Through their software accelerator RAPIDS, NVIDIA demonstrated how the rapid construction of end-to-end data science pipelines and seamless integration with common APIs could result in efficient, high-performing solutions that are at the cutting edge of technology. This feat underscores NVIDIA’s significant contributions to the advancement of recommender systems and their commitment to driving innovation in the AI space.
A Deep Dive into Recommender Systems
Recommender systems, a distinct subset of AI tools, are increasingly powering the digital economy. They are intricately designed systems that leverage complex algorithms to sift through vast data sets, methodically examining user preferences, behaviors, and a multitude of other factors. By doing so, they generate tailor-made recommendations for products, services, or content that cater to the individual needs and preferences of the users. They perform pivotal functions across various sectors, from curating entertainment playlists to assisting in healthcare diagnoses, guiding investment decisions in finance, and much more. The mounting significance of these systems stems from their ability to enhance customer engagement, improve decision-making processes, and boost the overall efficiency of services.
Types of Recommender Systems: A Detailed Exploration
Recommender systems essentially rank or score a user’s interest in a set of items. However, this process is far more complex than it may appear due to various real-world constraints. NVIDIA proposes a 4-stage recommender system that aims to address these challenges:
Candidate Retrieval
With the items catalog potentially running into the billions, it’s not feasible to score every item for every user. To address this issue, a relevant subset of items is quickly selected for scoring. This stage, known as candidate generation, can employ several models, including matrix factorization, two-tower, linear models, approximate nearest neighbor, and graph traversal.
Filtering
There are certain items, even with a smaller set of items, that should not be shown to the user due to several reasons such as availability, age appropriateness, previous consumption, or licensing issues. A filtering stage, therefore, is incorporated to apply such business logic rules, which might otherwise be difficult to enforce by the model.
Scoring
A scoring model determines the level of interest the user might have in each item, providing a list of relevant recommendations and their corresponding scores.
Ordering
Recommendations are often presented as a list. The Ordering stage aligns the output of the model with other needs or business constraints, attempting to provide a diverse set of items to the user.
These four stages represent a common design pattern across most real-world recommender systems today.
Real-World Examples
The recommender system represented in the four stages just covered can be seen in the recommendation engine architecture of various well-known companies, including:
- Meta’s Instagram: Instagram uses the IGQL query language which precisely maps onto these four stages.
- Pinterest: Pinterest’s architecture evolution over time reveals the same pattern, albeit with retrieval and filtering merged into a single stage.
- Instacart: In 2016, Instacart shared a recommendation system architecture that also follows these four stages directly, ensuring diversity in the final set of results presented to the user.
These complex systems are much more than a single model, and building them can be overwhelming. NVIDIA’s recommender system framework, Merlin, seeks to address these complexities, ensuring robust solutions for the RecSys space. Understanding these real-world recommender systems can help bridge the gap between theory and application, ultimately benefiting both practitioners and end-users.
Addressing Challenges in Recommendation Systems
Despite their substantial benefits, recommendation systems face significant challenges, including data sparsity, the cold start problem, scalability issues with large datasets, overfitting, ensuring diversity, and privacy concerns. Each challenge demands specific solutions, such as matrix factorization techniques or collaborative filtering algorithms for data sparsity, content-based filtering for the cold start problem, distributed computing frameworks or caching for scalability issues, and regularization techniques and cross-validation to prevent overfitting. Ensuring diversity and addressing privacy concerns requires employing diversity metrics, serendipity-based recommendations, anonymization techniques, and differential privacy.
Session-based Recommendations: A Focused Approach
Session-based recommendations represent a focused approach within the broader sphere of recommendation systems. They mainly consider the sequence of actions a user performs within a single session, instead of relying on the user’s historical data. This approach is effective in addressing some of the challenges in recommendation systems, like the cold start problem, scalability issues, and certain privacy concerns.
Research Insights on Recommender Systems
Research offers various insights into the strengths and potential risks of recommender systems. The MIT Sloan Management Review suggests that while recommender systems guide consumer choices effectively and enhance sales, they can also shape and reinforce consumer preferences and biases. The JSTOR study illustrates that strong recommendations boost sales and enable retailers to manipulate prices and stimulate demand. The Brookings article underscores the role of recommender systems in disseminating information while also cautioning about potential dangers such as promoting hate speech and political extremism.
Looking Ahead
As we look to the future, recommender systems are expected to continue evolving, providing accurate and efficient decision making. Hybrid models integrating various recommendation methods and possibly human judgement might become prevalent. As the volume of data increases and users seek increasingly personalized experiences, the need for systems capable of efficiently managing large data volumes while respecting privacy will only grow.
By Ronald van Loon